The VIBEs Team’s research in integrated Structural Health Monitoring and Bridge Weigh in Motion earned Amin Moghadam an International Society of Weigh in Motion (ISWIM) Young Researcher Award and featured in their December, 2020 Newsletter.
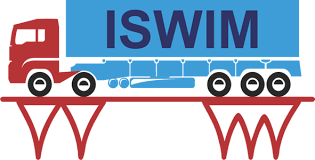
The VIBEs Team’s research in integrated Structural Health Monitoring and Bridge Weigh in Motion earned Amin Moghadam an International Society of Weigh in Motion (ISWIM) Young Researcher Award and featured in their December, 2020 Newsletter.
VIBEs Lab PhD Student Amin Moghadam featured in the Virginia Tech Civil and Environmental Engineering website for his research and acheivements. Full Article Here: https://cee.vt.edu/News-Menu/CEE_Article_Cache/Instrumentedbridges.html
Rodrigo Sarlo, Alan Smith, and Mohammad-Hesam Soleimani-Babakamali
One of the current challenges in structural health monitoring (SHM) is the design of methods that are flexible enough to scale to a variety of as-built structures. Current methods have limited scalability partly because they tend to rely on significant a priori information, e.g., an assumed model/geometry of the structure, training examples of specific damage cases, etc. Not only is such information sometimes difficult to obtain, it also results in a number of specific challenges that will be postulated as part of this talk. These include: 1) deviations of the as-built behavior from the assumed “as-planned” behavior and 2) limits on an SHM algorithm’s ability to deal with new or evolving damage or environmental scenarios. Based on these challenges, the talk will introduce a set of on-line monitoring methodologies which aim to reduce reliance on a priori information while accounting for the numerous variations present in the true built environment. The first challenge is addressed through the conversion of dense reality capture date (e.g., from LiDAR) to workable structural models, enabling the analysis of as-built geometries that evolve over the structure’s lifetime. The second challenge is addressed via an unsupervised SHM framework which exploits simple, high-dimensional features in combination with generative adversarial networks (GANs). Synthetic data from the GANs is analyzed using system reliability principles in order to adaptively define damage detection thresholds completely online. Both frameworks will be demonstrated on a variety of full-scale experimental structures to support their scalability potential.
Dr. Sarlo received the Virginia Tech TLOS XCaliber award for his integration of 3D printed bridge design into CEE 3404: Intro to Structures. https://vtx.vt.edu/awards/facstaff2021-1/bio-pages.html
The VIBEs Lab team pitch for gait diagnosis via floor vibrations (FloorVibe) was selected as one of 11 finalists for the 2021 Smart City Works Smart City Challenge. See the pitch here!
Rodrigo Sarlo and Rafael Gonçalves
Frailty is characterized by the functional decline of various physiological systems and is strongly associated with an increased vulnerability to stressors, such as illnesses or surgical procedures. Risk of frailty increase as we age. However, due to its complex influences, identifying frailty is challenging. Common methods, often based on surveys or visual observations, can vary by as much as 4 to 59% for similar populations of older adults. Recent research on older adults suggest that gait can serve as a predictor of frailty and associated adverse outcomes, such as reduced mobility, functional dependence, and mortality. In this presentation, we describe our efforts to build deployable, sensor-based techniques for monitoring several dimensions of gait. These techniques are passive, as they do not monitor the patient directly. This design is intended to create less privacy and intrusion concerns for older adults, thus improving the potential for adoption.
Dr. Rodrigo Sarlo, Virginia Tech
Hosted by: Soto Structures Lab (www.sotostructures.com)
The growing popularity of concepts like Smart Cities, Internet-of-things, and Digital Twins means we are collecting more sensor data on infrastructure than ever before. However, raw data alone has little value, what is more important is the information it provides and the decisions that it enables. Certain types of sensing modalities (e.g. accelerometers, cameras, and LIDAR) collect high resolution data that can yield a variety of rich, and sometimes unexpected, information. Through targeted signal processing and analysis, it is possible to infer high-level information for a variety of civil infrastructure applications. “Inference” is the key word, since much of the information contained in physical data is not readily obvious from observation alone. Our lab is multi-modal inference techniques which produce high-level, actionable data, including: 1) Using LIDAR point clouds to infer a building’s structural design, 2) Using traffic cameras to infer structural damage in traffic light masts, 3) Using vibrations to infer building occupancy patterns for operation management. Each of these examples demonstrate one of three main advantages of multi-modal inference techniques. Firstly, they value to certain data acquisition techniques by making them more versatile. Secondly, they are useful for “recycling” existing infrastructure data for a new purpose. Finally, they can be used to design infrastructure hardware which adapts to future needs.